The evolution of software testing is accelerating with the rise of AI-powered testing tools and automated testing software. As software quality becomes a top priority in modern SDLC methodologies, organizations are turning to AI and machine learning to streamline testing processes.

From automated functional testing to automated API testing, these innovations are reshaping QA software testing in outsourcing environments. With advanced machine learning models and generative AI testing tools, the future of software quality assurance looks smarter, faster, and more scalable. This blog explores how AI-driven test strategies are transforming software testing services worldwide.

What’s next? Keep scrolling to find out:
🚀 AI-Powered Testing Tools: Revolutionizing outsourced QA with improved speed, accuracy, and cost-efficiency.
🚀 Automated Testing Rise: Scaling global outsourcing with reduced human error and increased efficiency.
🚀 Elevating QA with AI: Smarter test strategies through AI-driven decision-making and risk prioritization.
🚀 Data Privacy in AI Testing: Addressing challenges in protecting sensitive data with AI-powered testing.
🚀 Choosing the Right Framework: Ensuring seamless, scalable, and cost-efficient frameworks for outsourced teams.
AI-Powered Testing Tools Reshaping Outsourced QA Services
Adopting AI-powered testing tools transforms how global teams approach outsourced QA software testing. These tools, built on machine learning and automated testing frameworks, are driving faster releases, deeper test coverage, and smarter bug detection.

- Speed: Automated functional testing accelerates test execution without compromising accuracy.
- Learning: Machine learning tools analyze past test data to predict future failures and optimize testing paths.
- Intelligence: AI machine learning enables self-healing test scripts that adapt to application changes.
- Scalability: Automated API testing ensures reliable backend validation across growing microservices.
- Integration: Software testing company services now include seamless integration of AI into the SDLC life cycle.
- Efficiency: Automated testing tools reduce testing time across multiple environments and platforms.
- Support: Many teams invest in software testing course programs to stay updated with evolving AI-based QA trends.
The Rise of Automated Software Testing in Global Outsourcing
The demand for faster releases and high-quality products has fueled the rise of automated software testing in global outsourcing. As organizations adopt modern SDLC methodologies, automation becomes essential to keep pace with agile development cycles.
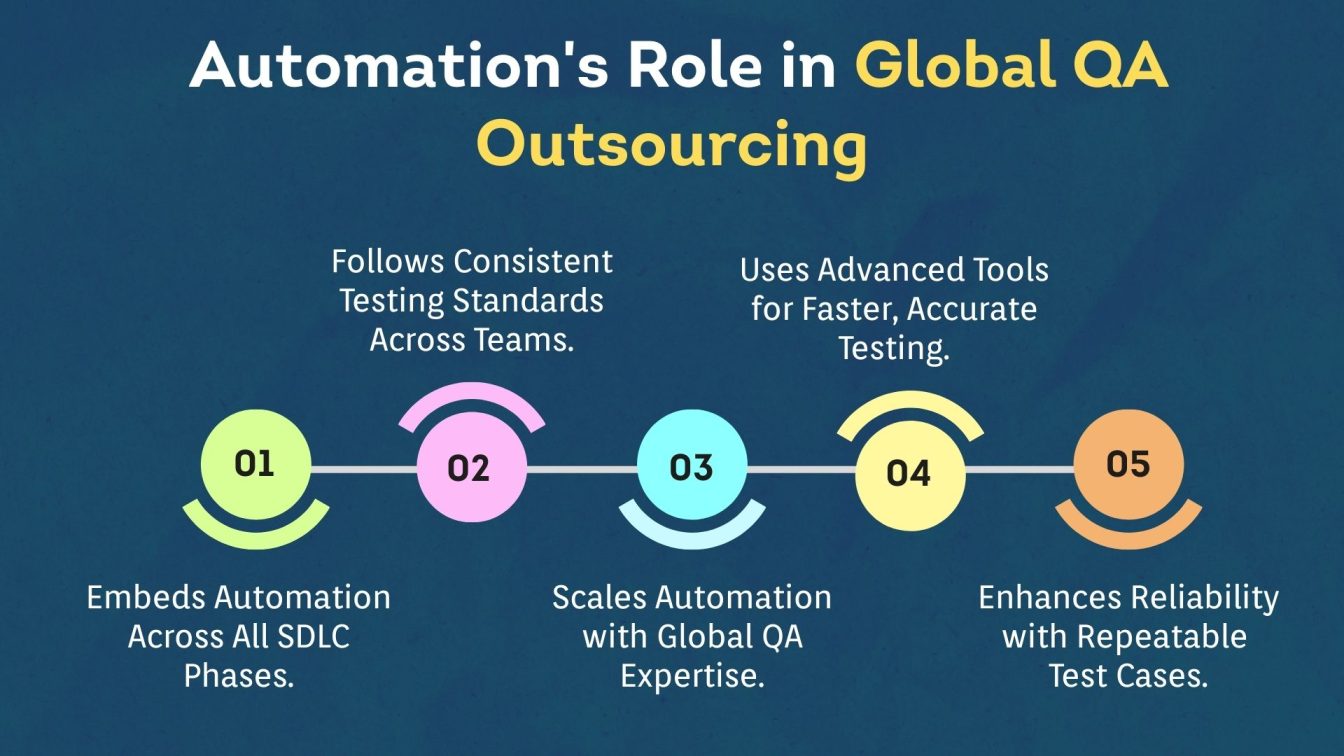
- Process Integration: SDLC in software engineering now embeds automation at every testing phase for better traceability.
- Standardization: Outsourced teams follow defined SDLC phases to ensure consistent testing practices across distributed teams.
- Capability Expansion: A reliable software testing company can scale automation efforts quickly using global QA talent.
- Tool Utilization: Outsourced partners utilize advanced automated testing tools for faster feedback and minimal manual errors.
- Reliability: Software quality testing improves through stable, repeatable automated test cases.
- Performance Focus: Integrated software performance testing ensures applications remain responsive under load, even in diverse deployment environments.
Elevating QA Outsourcing Through AI-Driven Test Strategies
The integration of AI tools for software testing is elevating how outsourced QA teams design and execute testing strategies. By embedding automated machine learning into testing pipelines, companies achieve more predictive and context-aware validation across the SDLC testing lifecycle.
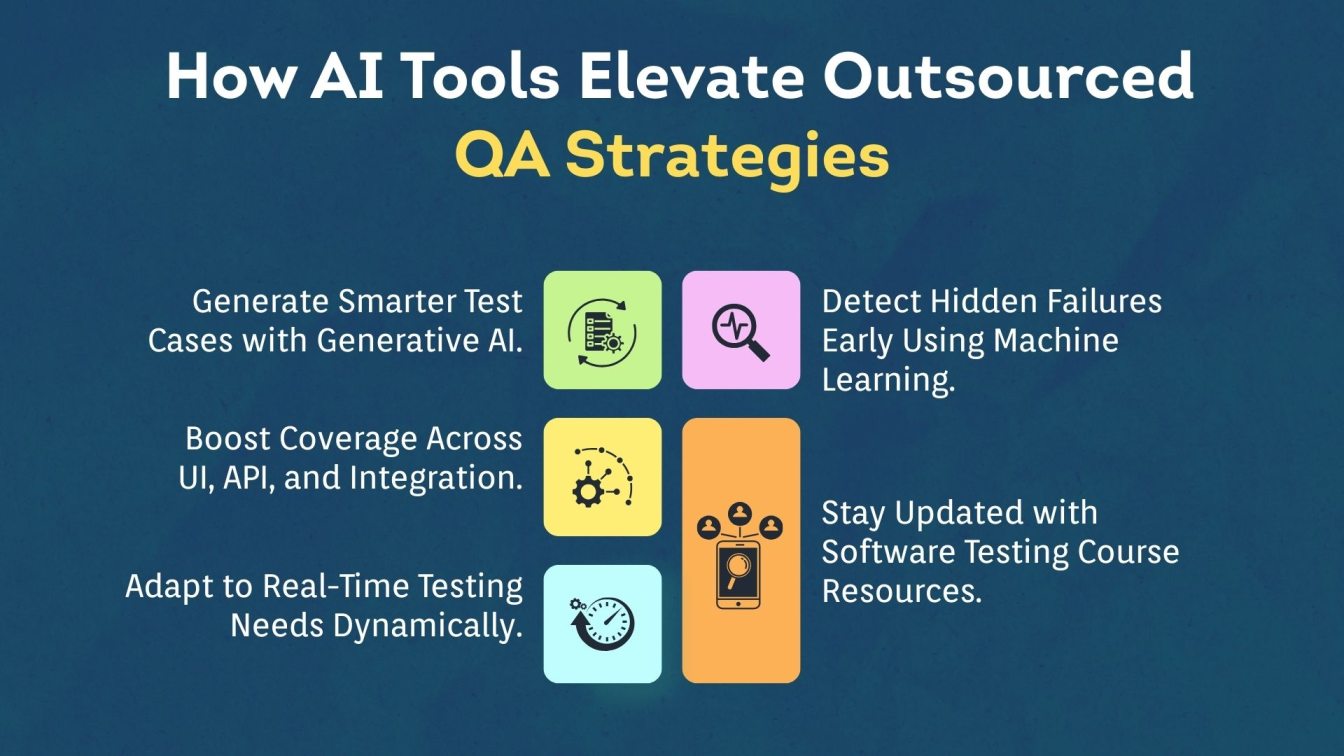
- Innovation: AI-powered testing tools leverage machine learning models to uncover hidden patterns and potential failures early.
- Agility: Outsourced QA providers use generative AI testing tools to quickly generate intelligent test cases.
- Learning Curve: Training programs and resources like software testing course modules help global QA teams stay AI-ready.
- Adaptability: AI and machine learning approaches allow real-time adjustments to shifting test requirements.
- Coverage: AI tools for software testing improve testing across UI, API, and integration layers.
- Advancement: Combining AI automation testing tools with cloud platforms enhances collaboration between development and QA teams.
Adaptive Test Maintenance Using AI in Outsourced Environments
Maintaining test scripts in fast-changing development cycles is a challenge for global teams. With the help of AI testing tools, outsourced environments are adopting adaptive test maintenance that reduces manual rework and ensures stability across releases.

- Self-Healing: AI automation testing tools automatically update broken test cases caused by UI or logic changes.
- Efficiency: Automated testing software reduces maintenance time by dynamically adjusting to new application flows.
- Learning-Driven: Machine learning algorithms detect patterns in failures and suggest targeted updates to test suites.
- SDLC Alignment: SDLC methodologies in outsourced models now prioritize AI-backed test optimization strategies.
- Error Reduction: AI tools for testing identify redundant test steps and recommend removals or improvements.
- Consistency: With continuous learning from test executions, automated testing services maintain consistency across evolving codebases.
Using AI to Align Testing Objectives with Business Goals in Outsourcing
Outsourced QA teams are now leveraging AI testing tools and strategic automation to better align testing efforts with core business goals. Through software quality assurance testing services, companies ensure that quality objectives directly support user expectations and release priorities.

- Goal Mapping: Quality assurance in software testing ensures every test case ties back to business functionality and value.
- Strategic Focus: Software quality assurance frameworks embedded with AI focus testing on high-risk, high-impact areas.
- Insight Generation: AI tools for testing provide dashboards and analytics for better decision-making and stakeholder communication.
- Outcome-Driven: Software quality is evaluated not just by defect count but by its effect on KPIs like customer retention and time-to-market.
- Unified Approach: Outsourcing partners align QA software testing strategies with broader software quality services for long-term success.
- Process Integration: Testing is now fully aligned with modern SDLC practices to ensure business-driven delivery.
How Outsourced Teams Use AI Tools to Ensure Continuous Quality
Outsourced testing teams are increasingly integrating AI-powered tools to drive consistent quality throughout the development cycle. These tools enhance test accuracy, reduce effort, and align seamlessly with agile workflows.

- Process Optimization: AI-driven testing enables smart decision-making based on predictive defect analysis.
- Faster Releases: Continuous testing ensures faster delivery without compromising on reliability.
- Reduced Overhead: Replacing time-consuming manual testing with intelligent automation lowers test maintenance costs.
- Efficiency Boost: Improved resource allocation allows teams to focus on critical and exploratory test areas.
- Speed & Accuracy: AI-driven tools help detect edge-case failures earlier in the SDLC.
- Team Collaboration: Enhanced coordination between development teams and QA speeds up bug resolution.
- Human-AI Synergy: Reducing human efforts on repetitive tasks increases focus on strategic validations.
- Business Impact: Delivering high-quality software within project timelines unlocks competitive advantages while minimizing false positives in defect detection.
- Agile Delivery: Supports continuous deployment in real-world outsourced testing team environments.
Why AI Integration Is a Competitive Edge for Outsourced Testing Vendors
Outsourced testing vendors are gaining a strong edge through strategic AI integration, reshaping how quality is achieved at speed and scale. This shift not only boosts operational efficiency but redefines the entire software development process.

- Scalability: AI systems can handle vast amounts of test data, identifying trends that manual methods overlook.
- Precision: Predictive analytics help prioritize high-risk areas, reducing escaped defects in production.
- Skill Synergy: AI augments human expertise, letting QA engineers focus on creative test design.
- Quality Focus: Vendors consistently deliver high-quality software that aligns with evolving business objectives.
- Efficiency: AI-driven testing tools reduce execution time while improving accuracy.
- Thorough Validation: Enables comprehensive testing across platforms and devices with less manual effort.
- Holistic Coverage: Ensures comprehensive test coverage throughout the software development lifecycle.
- Performance Insight: AI tracks response times, simulates real user interactions, and enhances User Experience across releases.
Strategic Outsourcing Models Enabled by AI-Powered Testing
As AI reshapes quality assurance, outsourcing models are evolving from basic support systems to deeply integrated, value-driven partnerships. AI-powered testing enables smarter frameworks that enhance decision-making, execution, and long-term results.

- User Interface: AI improves UI validation, ensuring seamless experiences across devices and platforms.
- Code Quality: Automated inspections identify inconsistencies early, strengthening release reliability.
- Real-time Feedback: AI provides instant insights, helping teams adjust quickly during testing cycles.
- AI-driven Solutions: These solutions automate routine tasks, freeing teams to focus on critical testing areas.
- Script Maintenance: AI simplifies test script updates when application logic changes.
- Potential Defects: Predictive models highlight high-risk zones, preventing defects from reaching production.
- Customer Satisfaction: Fast, error-free releases result in better client relationships and customer experience.
- Improved Efficiency: Streamlined processes minimize costs and resource waste.
- Manual Testers: AI complements, not replaces, human resources, closing skill gaps with a data-driven approach and a proactive approach to quality.
Outsourcing vs In-House: Where AI Testing Tools Work Best
As AI testing tools become more sophisticated, companies are rethinking traditional QA approaches. Deciding between outsourcing and in-house teams now hinges on flexibility, resources, and how well organizations leverage automation and intelligence.
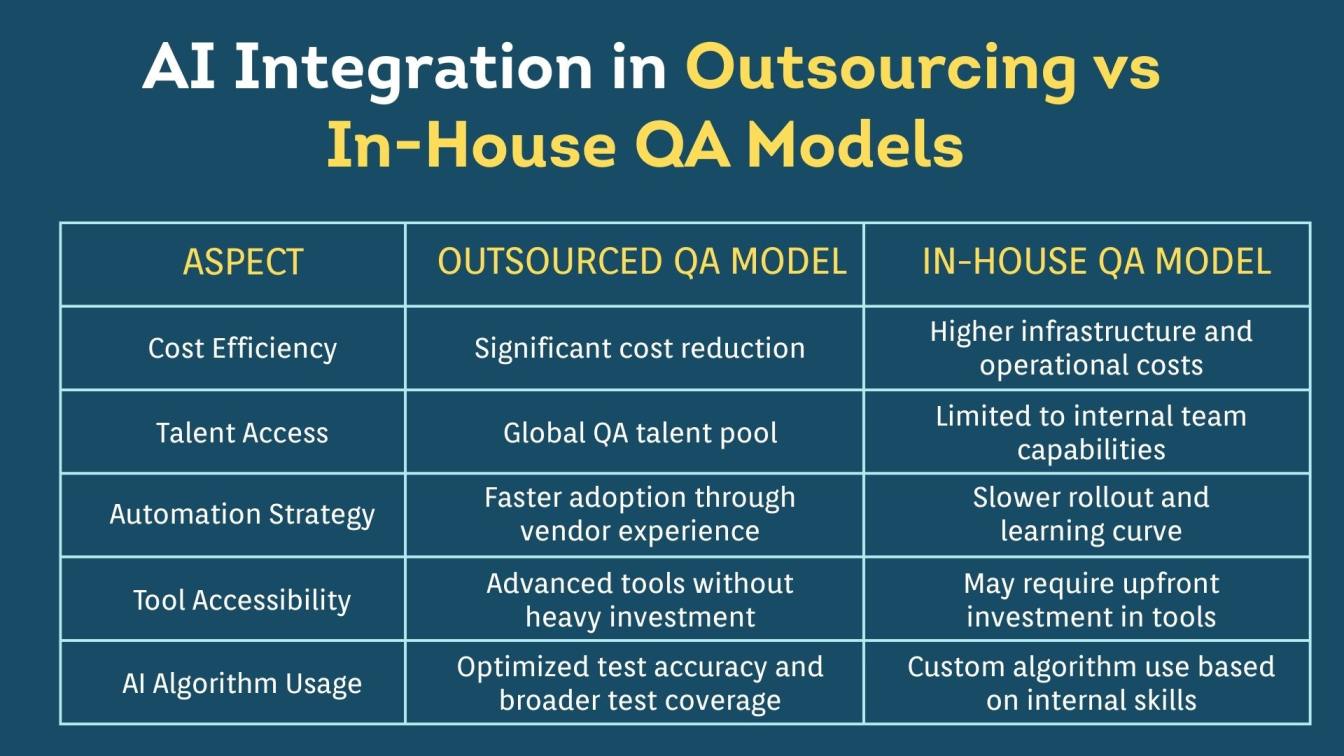
- Key Advantages: Outsourced models offer round-the-clock productivity, cost reduction, and access to global talent.
- Intelligent Algorithms: These algorithms improve test accuracy and optimize test coverage in both models.
- Automation Strategies: Outsourcing vendors often implement mature automation strategies faster than in-house teams.
- Advanced Tools: Access to advanced tools without heavy infrastructure investments makes outsourcing appealing.
- Remote Teams: Distributed remote teams ensure business continuity and scalable execution across time zones.
- Faster Testing: AI enables faster testing cycles, helping both setups release updates more frequently and reliably.
Data Privacy Concerns in AI-Driven Outsourced QA
As businesses adopt AI in quality assurance, outsourcing QA introduces serious data privacy concerns. When confidential information moves between global partners, safeguarding it becomes critical. Here's how data privacy can be compromised and what must be addressed:

- Data breach risks: Sharing data with third-party vendors using AI-based testing platforms increases vulnerability to unauthorized access or leaks.
- Compliance issues: Outsourced teams may operate in regions with different data protection regulations, making it hard to align with laws like GDP or CCPA.
- Training data exposure: AI tools require vast datasets, which can expose sensitive customer data if not properly anonymized during training processes.
- Lack of transparency: Without insight into how AI algorithms make decisions, companies risk failing to detect potential misuse of confidential information.
- Vendor reliability: Not all outsourcing vendors implement strong cybersecurity practices, leading to potential data misuse or unauthorized storage.
Choosing the Right Automated Testing Framework for Outsourced Teams
Outsourced QA teams need a robust automated testing framework to ensure speed, reliability, and collaboration across borders. Selecting the right one depends on compatibility, scalability, and integration with existing systems. Here are essential considerations:

- Framework compatibility: Ensure the framework supports the target operating systems, devices, and technologies used in the development environment.
- Cross-browser testing: A good framework allows seamless cross-browser testing to ensure consistent performance across Chrome, Firefox, Safari, and others.
- Integration support: Choose frameworks that integrate with popular CI/CD tools, bug tracking solutions, and project-management platforms.Faut
- Ease of use: Outsourced teams benefit from user-friendly interfaces and minimal setup, enabling faster onboarding and reduced manual intervention.
- Scalability: The chosen framework should scale with remote teams, enabling parallel execution and broader test coverage without compromising performance.
- Cost-efficiency: Frameworks should reduce costs while delivering actionable insights and measurable testing outcomes.
Conclusion..
AI-driven testing is reshaping outsourced QA services, boosting efficiency and quality. By leveraging automation and machine learning, teams can accelerate testing, optimize resources, and ensure alignment with business goals. The strategic use of AI tools offers a competitive advantage, enabling faster, more reliable software delivery. Embracing these innovations positions organizations to thrive in an increasingly digital and fast-paced environment.
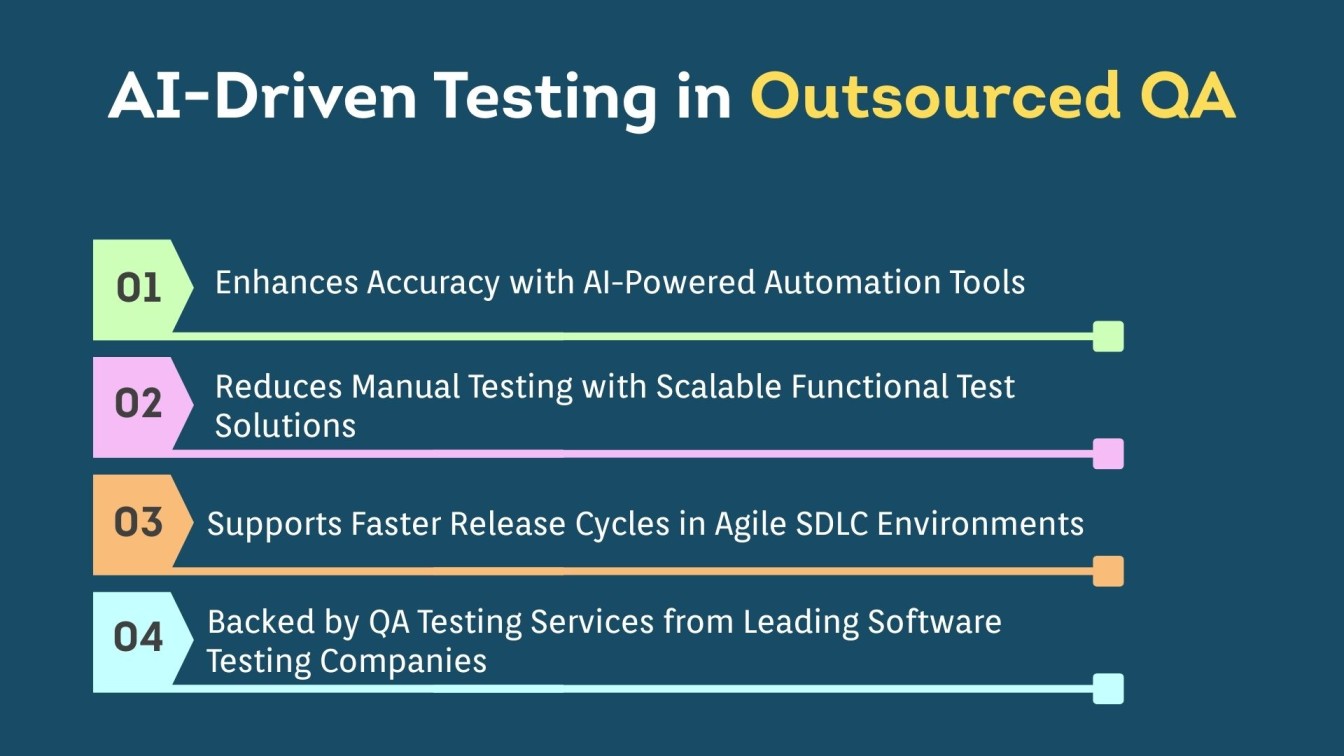
AI-driven test automation services are transforming outsourced QA by enhancing efficiency and accuracy. With tools like selenium automation testing service and functional test solutions, teams can scale testing efforts while reducing manual work. Leading software testing companies offer specialized QA testing services for enterprises, ensuring faster release cycles and superior software quality. This shift is revolutionizing how QA teams approach testing, aligning with modern SDLC needs for faster, more reliable results.
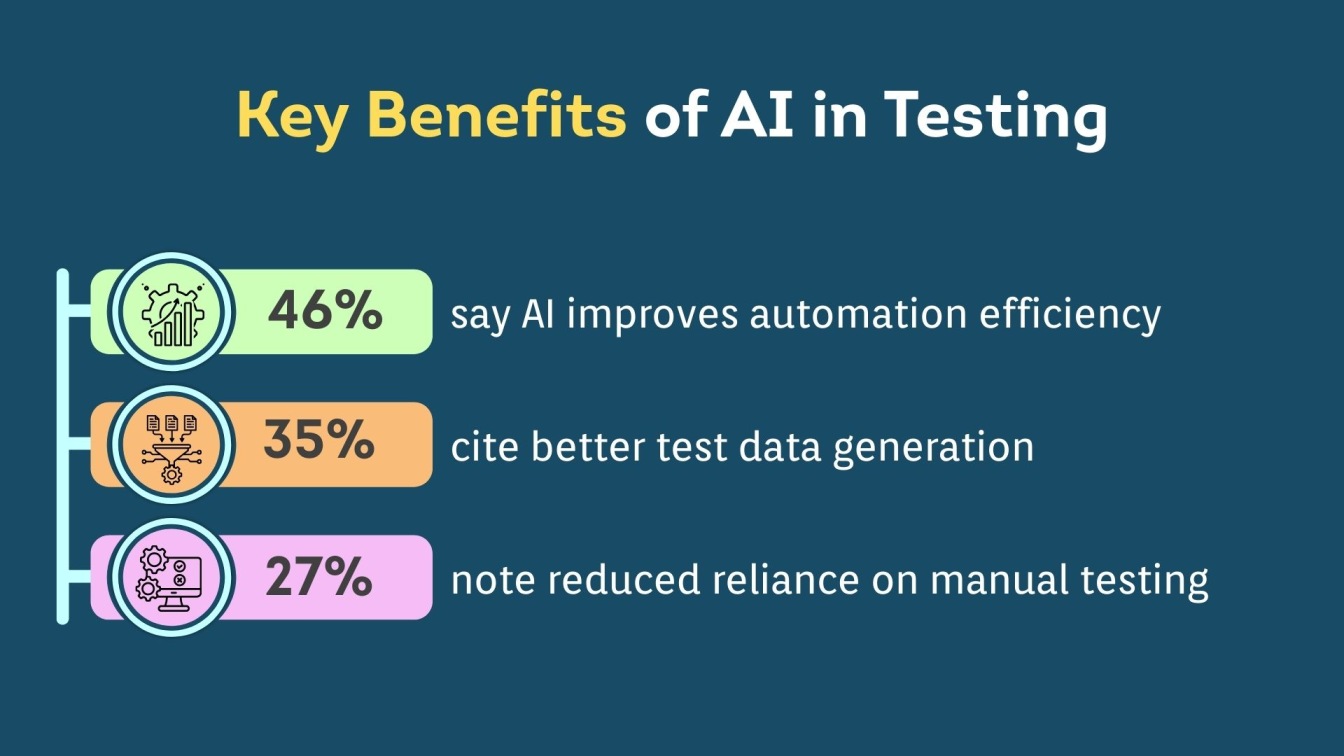
People Also Ask
What is the difference between AI and automation in software testing?
Automation follows predefined rules for repetitive tasks, while AI learns from data to make intelligent decisions and adapt during testing.
What are the limitations of using AI in automation testing?
AI struggles with context understanding, test case creativity, and dynamic UI changes and lacks human intuition in exploratory testing.
Which types of software tests are still difficult to automate with AI?
Exploratory, usability, visual validation, and complex end-to-end tests involving human judgment remain challenging for AI.
Is quality assurance (QA) at risk of being replaced by AI tools?
No, AI enhances QA but cannot fully replace human insight, critical thinking, and domain expertise in software testing.
How does AI impact decision-making in the QA process?
AI supports decision-making with predictive analytics, faster test prioritization, and defect pattern recognition to guide QA strategies.