In the dynamic and ever-changing digital world, businesses are constantly seeking innovative solutions to enhance efficiency and ensure high-quality software delivery. AI is an incredible force that is revolutionizing the realm of test automation. 🚀✨ By harnessing the power of artificial intelligence, organizations can streamline their testing processes, minimize human error, and significantly accelerate their time-to-market.
In this blog, we'll delve into:
- Understanding AI in Test Automation 🤖
Explore how AI technologies are integrated into testing frameworks, enhancing traditional methods. - Benefits of AI-Driven Testing 🏆
Discover the key advantages of implementing AI, including improved accuracy, efficiency, and scalability in testing processes. - Real-World Examples 🌍
Review compelling case studies that showcase successful AI implementations in test automation across various industries. - Challenges and Considerations ⚖️
Identify potential pitfalls and best practices to navigate the transition to AI-driven testing. - The Future of Testing with AI 🔮
Gain insights into emerging trends and predictions that will shape the future landscape of test automation.
Join us as we explore the transformative power of AI and its profound impact on test automation and overall business efficiency!
The Evolution of Test Automation with AI
The Ecosystem of test automation has undergone significant changes over the years, and the introduction of AI has been a key catalyst in this evolution. Let’s explore how AI is reshaping test automation and what this means for the future! 🌟
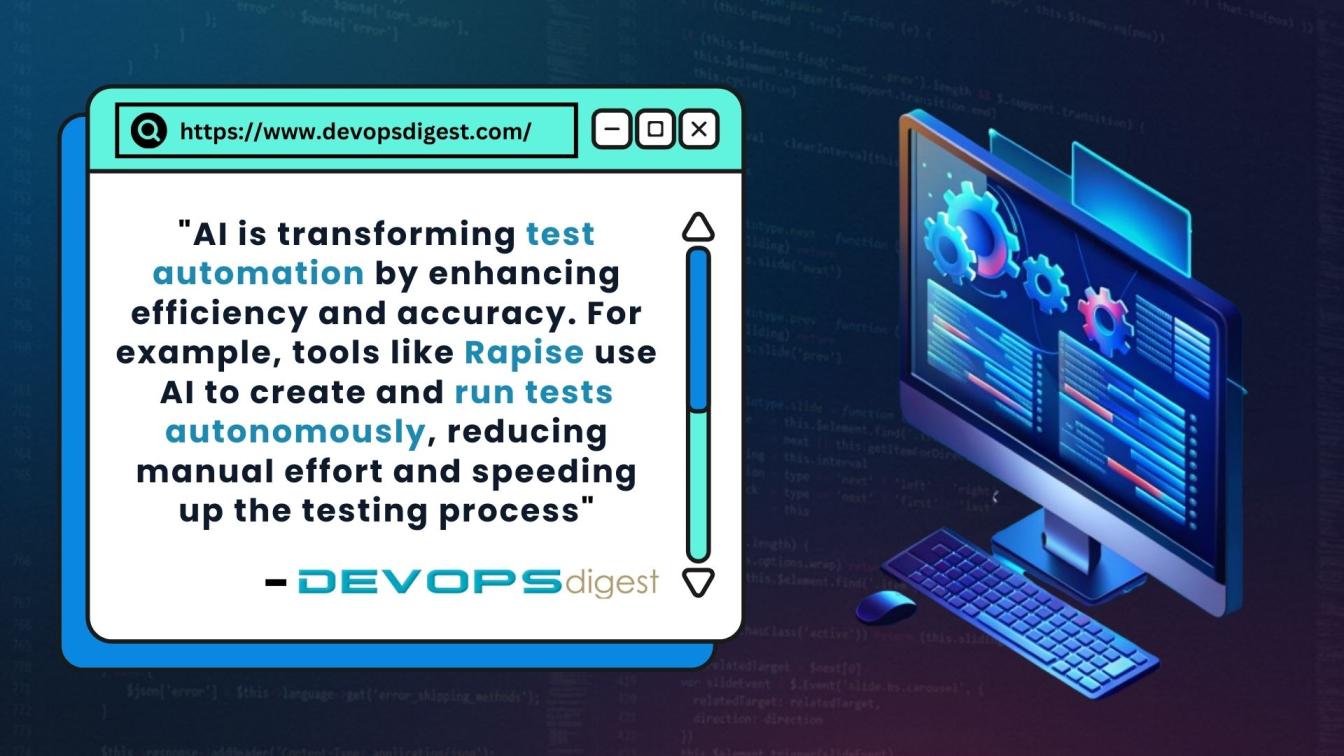
1. From Manual Testing to Automation 🔍➡️🤖
Initially, software testing was predominantly a manual process, relying heavily on human effort to execute test cases and report defects. This approach, while thorough, was time-consuming and prone to errors. The advent of automation tools marked the beginning of a new era, allowing repetitive tasks to be performed with greater efficiency and accuracy.
2. The Rise of Smart Automation 🧠💻
With the integration of AI, test automation has become "smart." AI algorithms can analyze vast amounts of data, learn from past test executions, and make intelligent decisions on what to test next. This proactive approach significantly reduces the time required for regression testing and enhances overall testing efficiency.
3. Enhanced Test Coverage and Quality Assurance ✅📈
AI-driven testing tools can automatically generate test cases based on user behavior and application usage patterns. This leads to improved test coverage and helps identify edge cases that might be overlooked in traditional testing. As a result, the quality of software products is enhanced, reducing the likelihood of defects in production.
4. Continuous Testing in DevOps 🚀🔄
The rise of DevOps practices has pushed for continuous testing, where testing is integrated throughout the development lifecycle. AI supports this by enabling faster feedback loops and automating testing in CI/CD pipelines. This integration allows teams to deliver high-quality software at a much faster pace.
5. Predictive Analytics for Risk Management 🔮📊
AI can analyze historical test data to predict potential risks and areas of concern in the software. By leveraging predictive analytics, organizations can prioritize testing efforts based on risk factors, ensuring that critical areas are thoroughly tested before release.
Benefits of AI-Driven Test Automation for Businesses
Integrating AI into test automation brings a multitude of benefits that can significantly enhance business operations. Here are some key advantages:
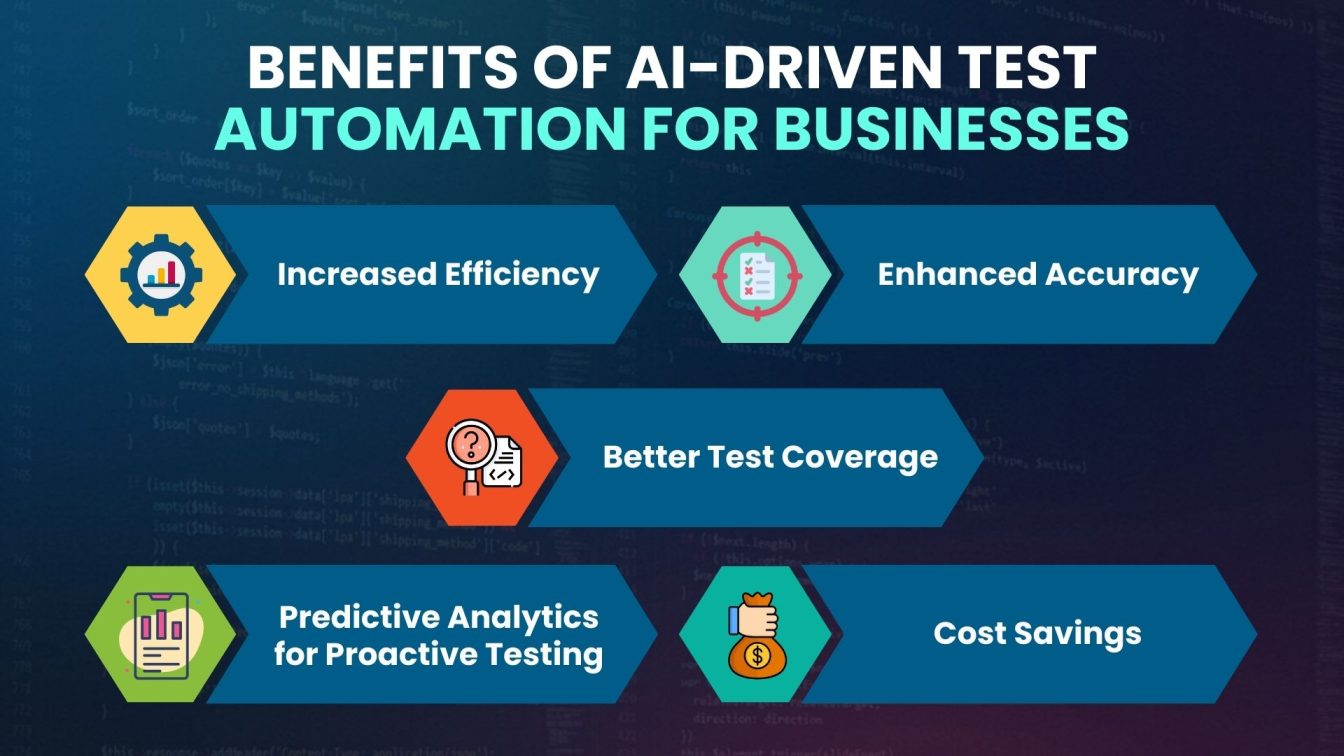
1. Increased Efficiency ⚡️
AI-driven tools can execute tests much faster than manual processes. They can run multiple tests simultaneously, drastically reducing the time needed for testing cycles. This allows teams to focus on more strategic tasks, speeding up the overall development process.
2. Enhanced Accuracy 🎯
AI minimizes the risk of human error that often occurs in manual testing. With intelligent algorithms analyzing code and execution paths, the accuracy of test results improves, leading to higher-quality software products.
3. Better Test Coverage 📈
AI can analyze user behavior and application usage patterns to generate comprehensive test cases. This ensures that edge cases and potential failure points are identified and tested, resulting in more robust applications.
4. Predictive Analytics for Proactive Testing 🔮
By leveraging historical data, AI can predict areas of high risk or likely defects. This allows teams to prioritize testing efforts on critical features, ensuring that potential issues are addressed before they reach production.
5. Cost Savings 💰
Automating tests with AI reduces the need for extensive manual testing resources. This can lead to significant cost savings in terms of time and personnel, ultimately enhancing the return on investment (ROI) for testing efforts.
Machine Learning Algorithms for Automated Test Case Generation
The integration of machine learning (ML) into automated test case generation is transforming the software testing landscape. By leveraging algorithms that can learn from data, businesses can create more effective and comprehensive test cases. Here’s a look at some key machine learning algorithms used for this purpose:
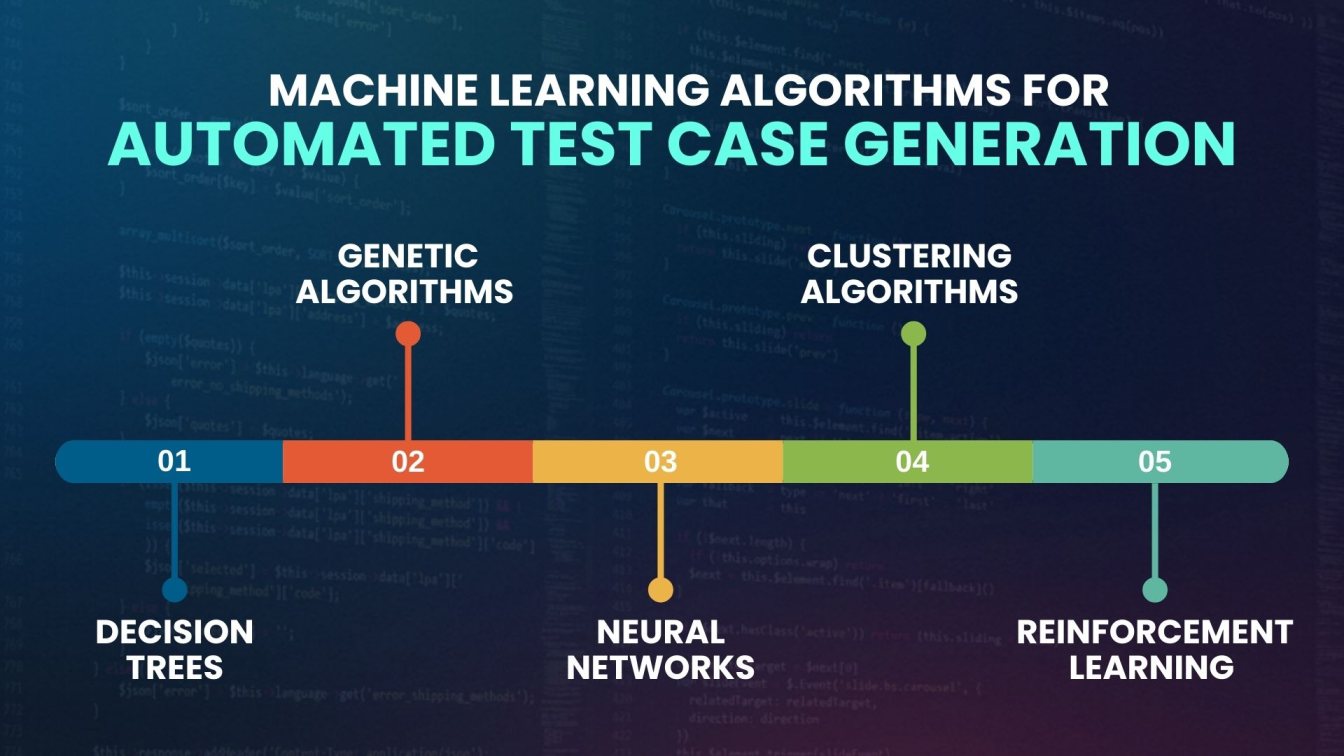
- Decision Trees 🌳
Decision trees are a popular choice for generating test cases due to their simplicity and interpretability. They model decisions based on a series of questions, making it easier to identify different paths through an application. By analyzing historical test data, decision trees can suggest new test cases that explore untested paths.
- Genetic Algorithms 🧬
Inspired by the process of natural selection, genetic algorithms can optimize test case generation by evolving a population of test cases over time. They assess the effectiveness of existing cases and combine or mutate them to create new, more effective tests. This approach can uncover hidden defects by exploring various combinations of inputs and execution paths.
- Neural Networks 🧠
Neural networks, particularly deep learning models, can analyze large datasets to identify patterns that might not be apparent through traditional testing methods. They can be trained to recognize which areas of an application are more prone to defects, thereby generating targeted test cases that focus on high-risk areas.
- Clustering Algorithms 📊
Clustering algorithms group similar data points, which can help identify patterns in user interactions with software. By understanding how different features are used, these algorithms can generate test cases that cover a wide range of usage scenarios, ensuring comprehensive testing.
- Reinforcement Learning 🎮
Reinforcement learning involves training an agent to make decisions based on rewards and penalties. In the context of test case generation, this approach can be used to create a feedback loop where the system learns which test cases are most effective and adjusts its strategy accordingly, optimizing the testing process over time.
How AI Enhances Test Coverage and Accuracy
Artificial Intelligence (AI) is revolutionizing the way test coverage and accuracy are approached in software testing. By leveraging advanced algorithms and data analytics, AI can significantly improve both the breadth and depth of testing processes. Here’s how:

- Smart Test Case Generation 📄
AI can analyze application code, user behavior, and historical data to automatically generate test cases that cover a wide range of scenarios. This smart generation ensures that critical paths and edge cases are tested, leading to more comprehensive test coverage.
- Behavioral Analysis 🔍
By employing machine learning algorithms, AI can study user interactions with the application to identify common patterns and potential areas of concern. This behavioral analysis allows for the creation of test cases that reflect real-world usage, ensuring that the most relevant scenarios are covered.
- Continuous Learning 📚
AI systems can learn from previous test results and adapt their strategies accordingly. By continuously analyzing data from past tests, AI can identify gaps in coverage and focus on areas that are prone to defects, enhancing both accuracy and effectiveness over time.
- Predictive Analytics 🔮
AI can utilize historical data to predict which features are most likely to fail based on usage patterns and defect history. This predictive capability allows teams to prioritize testing efforts, ensuring that high-risk areas receive adequate attention, thus improving overall accuracy.
- Automated Regression Testing 🔄
AI can efficiently manage regression testing by automatically identifying changes in the codebase and determining which tests are affected. This targeted approach reduces redundant testing and focuses on areas most likely to introduce errors, enhancing both coverage and accuracy.
Reducing Testing Time and Costs with AI
In the competitive ecosystem of software development, efficiency is paramount. AI-driven testing strategies are proving to be invaluable in minimizing both testing time and costs, enabling organizations to deliver high-quality software faster and more economically. Here’s how AI achieves these significant reductions:
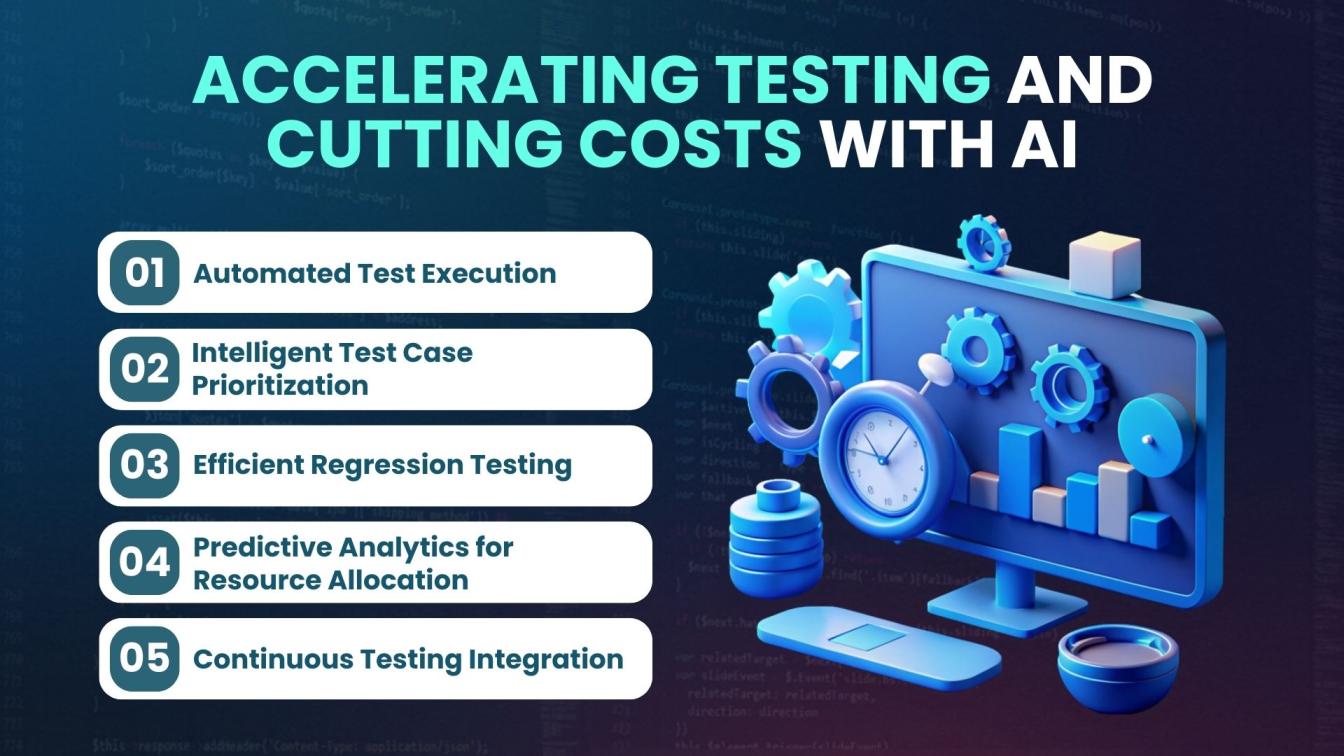
1. Automated Test Execution ⚙️
AI tools can automate repetitive testing tasks, significantly reducing the manual effort required. Automated tests can run 24/7, allowing for faster execution of test cases without the limitations of human resources, which leads to quicker feedback cycles.
2. Intelligent Test Case Prioritization 📊
AI can analyze historical test data to determine which test cases are most critical based on risk and impact. By prioritizing high-risk areas, organizations can focus their efforts on the tests that matter most, reducing overall testing time and ensuring critical features are thoroughly vetted.
3. Efficient Regression Testing 🔄
Regression testing is essential for maintaining software quality, but it can be time-consuming. AI can intelligently select and execute only the necessary regression tests based on recent code changes, minimizing the time spent on tests that are unlikely to reveal new defects.
4. Predictive Analytics for Resource Allocation 🔮
By utilizing predictive analytics, AI helps organizations allocate resources more effectively. By predicting which areas of the application are likely to encounter issues, teams can direct their testing efforts where they are needed most, optimizing resource use and reducing wasted effort.
5. Continuous Testing Integration 🚀
AI facilitates continuous testing within CI/CD pipelines, ensuring that tests are executed in real-time as code changes are made. This integration allows for immediate feedback, preventing delays caused by late-stage testing and reducing the time to identify and resolve issues.
AI-Powered Tools and Technologies in Test Automation

AI is transforming test automation through a range of powerful tools and technologies that enhance efficiency, accuracy, and coverage. Here are some notable AI-powered tools making waves in the industry:
1. Applitools
Employs visual AI to automate visual testing, ensuring that applications appear correctly across different devices and browsers.

2. Testim
Utilizes machine learning to create robust automated tests that adapt to changes in the application, significantly reducing maintenance time.

3. Mabl
An intelligent test automation tool that uses AI to automatically generate, execute, and maintain tests, allowing teams to focus on critical testing areas.

4. Functionize
Combines natural language processing and machine learning to enable users to write tests in plain English, simplifying the testing process for non-technical users.

5. Test.ai
Automates the creation of test cases by analyzing user interactions within applications, and generating tests that reflect real user behavior.
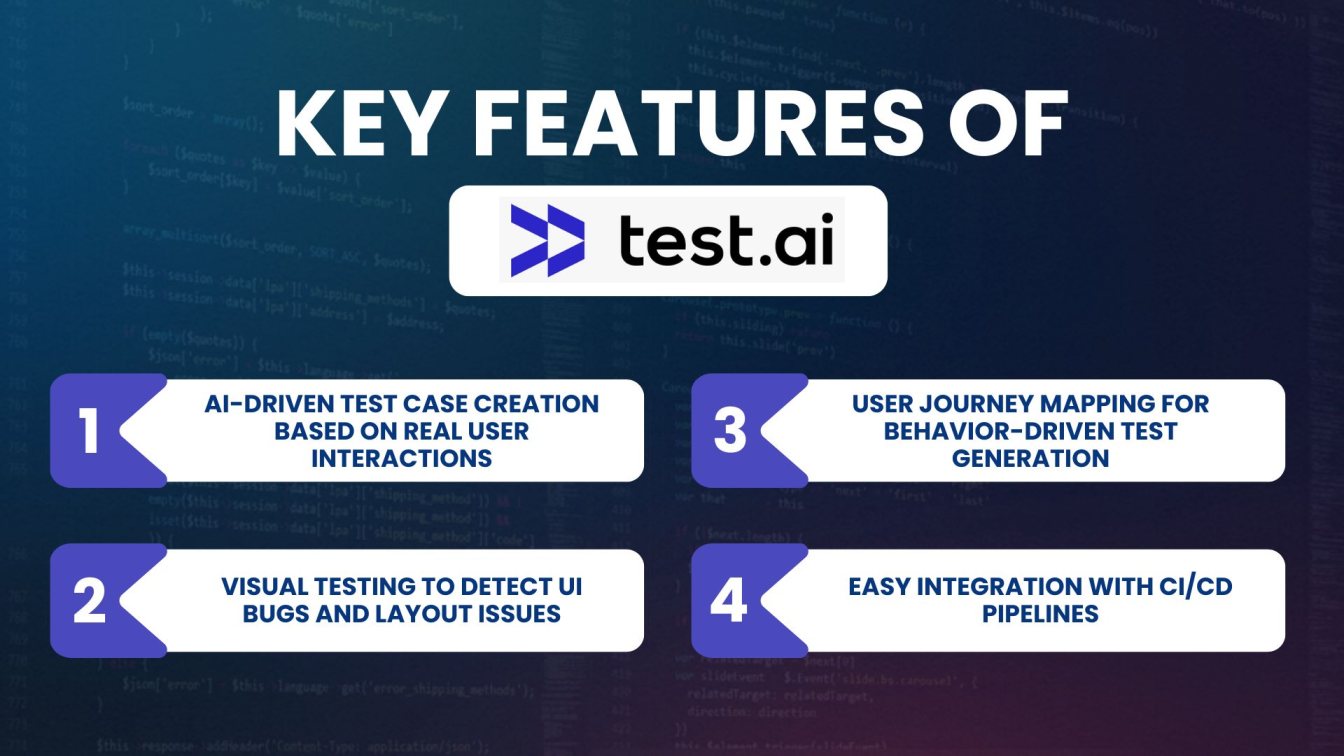
Overcoming Challenges in Implementing AI for Test Automation
Implementing AI in test automation can bring significant benefits, but it also comes with its own set of challenges. Here are key strategies to overcome these hurdles:
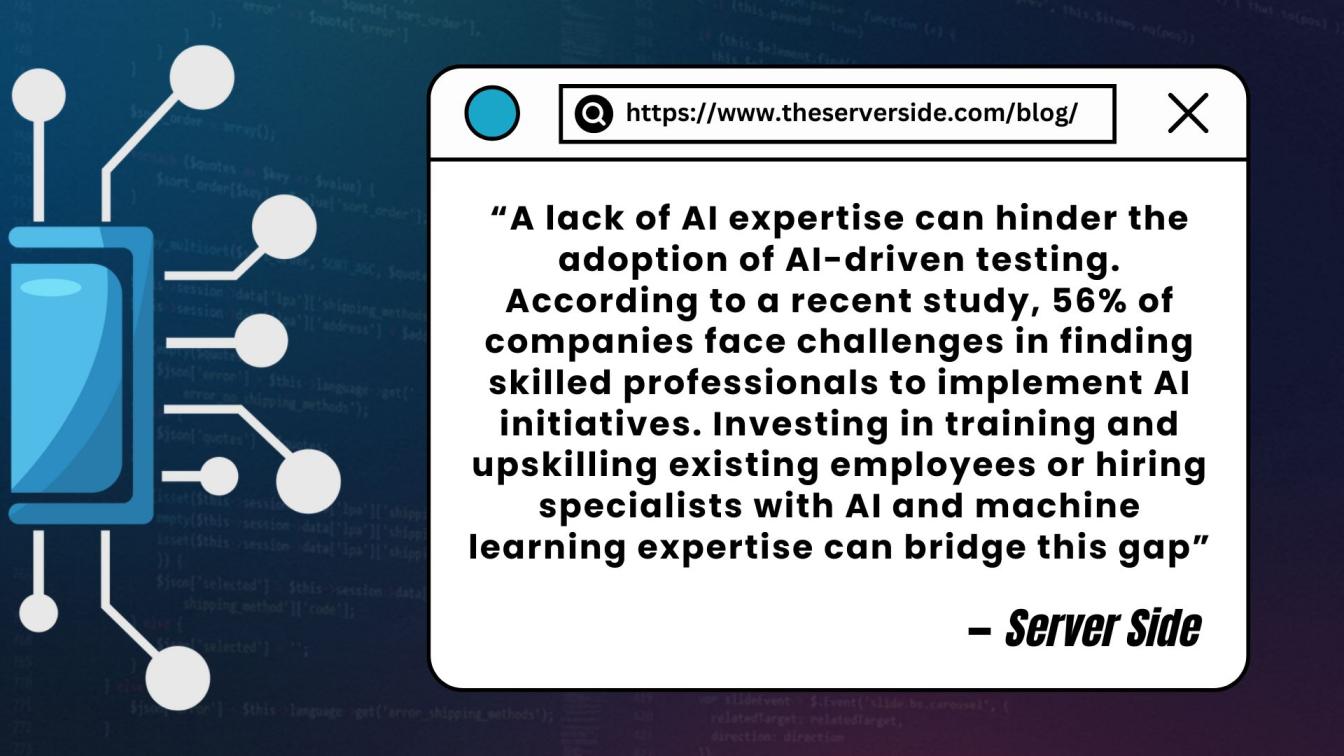
1. Data Quality and Availability 📊
Challenge: AI relies heavily on quality data for training and accuracy.
Solution: Ensure clean, well-organized datasets and utilize tools that can automatically collect and preprocess data for AI models.
2. Skill Gaps in the Team 🧑🏫
Challenge: Teams may lack the necessary skills to implement AI effectively.
Solution: Invest in training and upskilling employees or hire experts with AI and machine learning experience.
3. Integration with Existing Tools 🔗
Challenge: Integrating AI tools with current testing frameworks can be complex.
Solution: Choose AI solutions that offer robust integration capabilities with existing CI/CD pipelines and testing tools.
4. Change Management 📈
Challenge: Resistance to change can hinder AI adoption.
Solution: Foster a culture of innovation by communicating the benefits of AI and involving stakeholders in the transition process.
5. Maintaining Test Accuracy 🔍
Challenge: AI models may become outdated or inaccurate over time.
Solution: Continuously monitor and retrain AI models using new data to ensure they remain relevant and effective.
Best Practices for Integrating AI into Your Testing Strategy
Successfully integrating AI into your testing strategy requires careful planning and execution. Here are some best practices to ensure a smooth transition:

1. Define Clear Objectives 🎯
Establish specific goals for what you want to achieve with AI in testing. This could include reducing testing time, improving accuracy, or increasing test coverage.
2. Start Small and Scale Gradually 📏
Begin with pilot projects to test the effectiveness of AI tools. Evaluate results before scaling up to more complex applications, allowing for adjustments based on initial feedback.
3. Ensure Data Quality 📊
High-quality data is essential for AI success. Regularly clean and maintain datasets to ensure they are accurate and representative, which will enhance the performance of AI models.
4. Choose the Right Tools 🛠️
Select AI tools that align with your testing needs and integrate seamlessly with existing testing frameworks. Look for solutions that offer scalability and flexibility.
5. Train Your Team 🧑🏫
Invest in training programs to upskill your testing team in AI and machine learning concepts. Empower them to leverage these technologies effectively within your testing processes.
AI in Identifying and Fixing Defects Early
Early detection and resolution of defects are crucial for maintaining software quality and reducing costs. AI technologies are playing a pivotal role in streamlining this process. Here’s how AI contributes to identifying and fixing defects early in the development cycle:
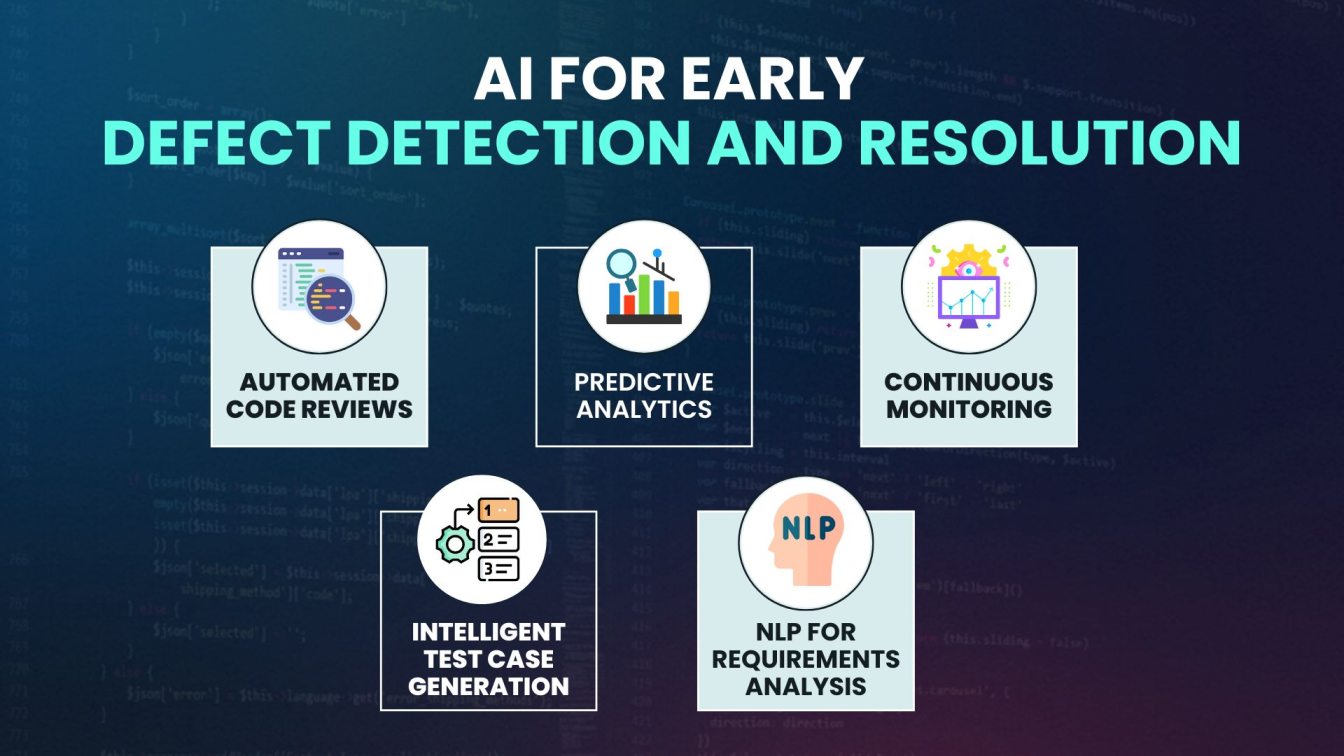
1. Automated Code Reviews 🔍
AI-powered tools can automatically review code for potential defects, analyzing syntax and coding standards. This proactive approach helps identify issues before they escalate, allowing developers to address them early.
2. Predictive Analytics 🔮
By leveraging historical data, AI can predict which parts of the code are most likely to contain defects based on patterns and trends. This targeted insight enables teams to focus their testing efforts on high-risk areas.
3. Continuous Monitoring 🕵️♂️
AI systems can continuously monitor applications during development and testing phases. By analyzing real-time data, they can quickly identify anomalies and potential defects, facilitating immediate action.
4. Intelligent Test Case Generation 📄
AI can generate test cases based on the code and user behavior, ensuring comprehensive coverage. By anticipating user interactions, AI helps uncover defects that might otherwise go unnoticed.
5. Natural Language Processing (NLP) for Requirements 📜
NLP can analyze requirements and user stories to identify inconsistencies or ambiguities that could lead to defects. By clarifying requirements early, teams can reduce the likelihood of defects related to misunderstandings.
Scalable Test Automation: How AI Makes It Possible
AI transforms test automation, enabling scalability in several impactful ways.
By dynamically generating test cases, AI ensures that testing keeps pace with evolving applications. It analyzes code changes and user behavior to create relevant tests automatically, allowing teams to adapt quickly as new features are added.

Parallel test execution is another critical advantage. AI tools can run tests across multiple environments simultaneously, significantly reducing the time required for testing. This capability is vital as test suites expand, ensuring timely feedback and quicker releases.
Intelligent test prioritization enhances efficiency further. AI assesses risk and historical data to determine which tests are most critical, ensuring that high-priority tests are executed first. This targeted approach maximizes resource utilization, especially in larger applications.
Maintenance becomes less burdensome with AI as well. Automated updates to test cases in response to application changes minimize manual effort, ensuring the test suite remains relevant without constant human intervention.
Lastly, continuous learning from testing data allows AI systems to refine their processes over time. This adaptability means that as testing requirements grow, AI-driven solutions become increasingly effective.
Quick Recap🌟
In conclusion, AI-driven scalable test automation empowers organizations to efficiently adapt their testing processes to meet the demands of evolving software. By dynamically generating test cases, executing tests in parallel, intelligently prioritizing efforts, and automating maintenance, AI not only enhances efficiency but also ensures high-quality standards. Embracing these technologies allows businesses to accelerate their development cycles and stay competitive in a rapidly changing landscape. 🌟
People also asked
👉How does AI improve the efficiency of test automation?
AI enhances efficiency by automating repetitive tasks, generating test cases dynamically, and enabling faster execution.
👉What are the key benefits of using AI in test automation for businesses?
Key benefits include increased test coverage, improved accuracy, faster feedback loops, and reduced manual effort.
👉What types of AI tools are commonly used in test automation?
Common AI tools include Testim, Applitools, Mabl, and Functionize, which facilitate automated testing and analysis.
👉Can AI-driven test automation reduce costs and time-to-market for software development?
Yes, AI-driven test automation can significantly reduce costs and time-to-market by streamlining testing processes and accelerating development cycles.
👉How does AI help in identifying and prioritizing test cases in automation?
AI analyzes historical data and risk factors to intelligently prioritize test cases, focusing on those most likely to uncover defects.
👉What are the challenges businesses might face when integrating AI into their test automation processes?
Challenges include data quality issues, skill gaps within the team, integration complexities, and resistance to change.